
There are already a handful of experimental projects, such as BabyAGI and AutoGPT, that hook chatbots up with other programs such as web browsers or word processors so that they can string together simple tasks.
#Download macjournal 5 how to
And if you want them to be good at it, you don’t want to micromanage them-you want them to figure out how to do it.” “Don’t think for a moment that Putin wouldn’t make hyper-intelligent robots with the goal of killing Ukrainians,” he says. What happens, he asks, when that ability is applied to something inherently immoral? Hinton believes that the next step for smart machines is the ability to create their own subgoals, interim steps required to carry out a task. They want to use them for winning wars or manipulating electorates.” “We know that a lot of the people who want to use these tools are bad actors like Putin or DeSantis. “Look, here’s one way it could all go wrong,” he says. He is especially worried that people could harness the tools he himself helped breathe life into to tilt the scales of some of the most consequential human experiences, especially elections and wars. I think they’re very close to it now and they will be much more intelligent than us in the future,” he says. “I have suddenly switched my views on whether these things are going to be more intelligent than us.
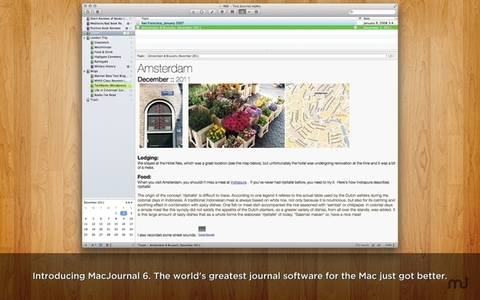
“It’s a completely different form of intelligence,” he says. What does all this add up to? Hinton now thinks there are two types of intelligence in the world: animal brains and neural networks. It’s as if there were 10,000 of us, and as soon as one person learns something, all of us know it.”
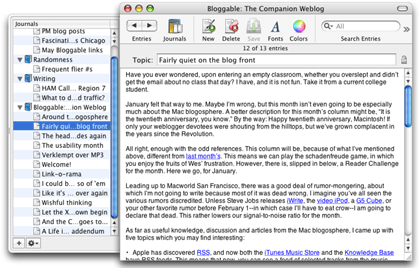
“But I can have 10,000 neural networks, each having their own experiences, and any of them can share what they learn instantly. “If you or I learn something and want to transfer that knowledge to someone else, we can’t just send them a copy,” he says. Learning is just the first string of Hinton’s argument. (And it's worth pausing to consider what those costs entail in terms of energy and carbon.) “When biological intelligence was evolving, it didn’t have access to a nuclear power station,” he says.īut Hinton’s point is that if we are willing to pay the higher costs of computing, there are crucial ways in which neural networks might beat biology at learning. And brains do it on a cup of coffee and a slice of toast. Of course, brains still do many things better than computers: drive a car, learn to walk, imagine the future. So maybe it’s actually got a much better learning algorithm than us.” Yet GPT-4 knows hundreds of times more than any one person does. “Large language models have up to half a trillion, a trillion at most. “Our brains have 100 trillion connections,” says Hinton. But they are tiny compared with the brain. But here’s his case.Īs their name suggests, large language models are made from massive neural networks with vast numbers of connections. Hinton’s fears will strike many as the stuff of science fiction. Now he thinks that’s changed: in trying to mimic what biological brains do, he thinks, we’ve come up with something better. And so it has to be possible to learn complicated things by changing the strengths of connections in an artificial neural network.” A new intelligenceįor 40 years, Hinton has seen artificial neural networks as a poor attempt to mimic biological ones. They’re doing it by changing the strengths of connections between neurons in their brain. They’re not doing it by storing strings of symbols and manipulating them.

“Crows can solve puzzles, and they don’t have language. “And symbolic reasoning is clearly not at the core of biological intelligence. “My father was a biologist, so I was thinking in biological terms,” says Hinton.
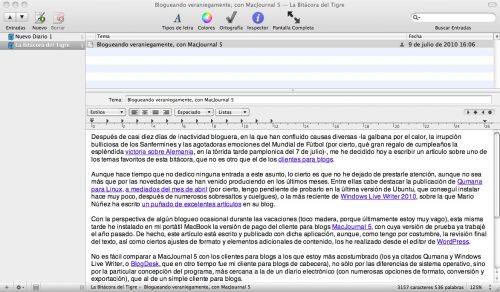
By changing how those neurons are connected-changing the numbers used to represent them-the neural network can be rewired on the fly.
#Download macjournal 5 software
He worked on neural networks, software abstractions of brains in which neurons and the connections between them are represented by code. The dominant idea at the time, known as symbolic AI, was that intelligence involved processing symbols, such as words or numbers.īut Hinton wasn’t convinced. “But it’s taken a long time to sink in that it needs to be done at a huge scale to be good.” Back in the 1980s, neural networks were a joke. “We got the first inklings that this stuff could be amazing,” says Hinton. One of these graduate students was Ilya Sutskever, who went on to cofound OpenAI and lead the development of ChatGPT. They also trained a neural network to predict the next letters in a sentence, a precursor to today’s large language models. Working with a couple of graduate students, Hinton showed that his technique was better than any others at getting a computer to identify objects in images. It took until the 2010s for the power of neural networks trained via backpropagation to truly make an impact.
